The Impact of Facial Characteristics on the Face-Naming Effect Through Human andAI-Generated Faces
- Levi Cottrell
- Feb 2
- 13 min read
Abstract
Previous research has shown a ‘face-naming’ effect by which humans and machine learning algorithms can guess the name of a face at an accuracy greater than random chance, given only 4-5 name options.This effect was previously studied in French and Israeli contexts. This study was conducted within amid-sized high school in a small town near Vancouver, BC, Canada, with 23 participants. This study comprised two sections. Participants were tested with human faces in the first section, with their real names, and 3 other names of similar popularity. An accuracy of approximately 19.48% was found in this experiment, where random chance is 25%. Participants were tested with AI-generated faces in the second
experiment, each with similar characteristics to a corresponding human face from the first experiment. These faces were tested with the same names as their similar human counterpart. An accuracy of approximately 17.8% was found in this experiment. This suggested that one: in this context, the face-naming effect did not emerge; and two: the face-naming effect is barely influenced by, if not hindered by, the similarity of facial characteristics in these AI-generated faces. The following study
explores this effect in detail.
Background
Names are an integral part of the human experience. The action of naming another person
originates from the need to refer to people as individuals instead of groups. Humans are not the only species that name each other; dolphins and elephants have complex social relationships, including naming systems. Elephants, for example, use specialized calls to address individuals.
Dolphins use a similar system, using signature whistles instead of calls to identify each other.
Humans, however, have an obsession with names, naming or finding the name of every item,
idea, and person they meet. Sociality is hypothesized to be the origin of this. Humans tend to personify different words and their sounds, usually based on safety and danger. For instance, softer phonemes (l, s, r, m, etc...) are usually common in the names for ‘softer’ items and ideas, whereas harsher phonemes (k, t, z, b, etc...) are generally found in relatively ‘harsher’ words.
Humans can predict the names of others at an accuracy higher than random probability
(Zwebner et al., 2017). This study aims to discover the existence of the face-naming effect in
differing contexts to those already explored and to find if facial characteristics impact said
face-naming effect. The study has two groups of participants, both given a demographic survey.The first group, or control group, was given a collection of photographs of humans, along with a list of four names, one of which was the person’s real name. The participants must match a name to the picture. The second group was given the same list of names, and a different set of similar faces generated by a GAN (generative adversarial network) AI model. After the collection of data, the results were compiled, possible biases and effects related to the face-naming effect were
extracted.
Review of Literature
Zwebner, Sellier, Rosenfield, Goldenberg, and Mayo (2017) studied the effects of social
perceptions on facial appearance, the ability of the general population to predict the names of faces, and the accuracy of computer algorithms in predicting the names of faces. They tested the hypothesis of a face-naming effect, where a person’s name shapes their facial appearance. Of their eight total studies, three are largely relevant to this study, including 1A, 1B, and 2.
In study 1A, a hypothesis-blind assistant obtained photos of Israeli young adults (20-30)
from a large social network platform. ‘Filler’ names were gathered from local websites
containing name catalogues. Four filler names were added to each of the photos along with the true names, in the manner of multiple-choice. The filler names did not match the true names and there was no repetition. In total, 80 filler names and 20 pictures with true names were selected. The order of images and name choices was random for each participant. Participants included one hundred twenty-one local Israeli students. (60 women, 61 men, all native speakers, M =age 27.77 years [SD 4.08]) Each participant was asked to choose one of the five names as the true name of the person depicted in the picture for a total of twenty faces. Results of the test where the participants were familiar with the face were discounted. The result had an average of 28.21% accuracy, where chance is 20%. This study established the existence of a possible face-naming effect.
Study 1B replicated study 1A with a larger sample of stimuli to refute the possibility that
the face-naming effect is due to name popularity. The study differed from the previous one
because filler names were gathered from the set of true names instead of local name catalogues.
In studies 1B and 2, photos were gathered through university campus announcements instead of online images and names. These pictures were taken front-on with a neutral expression at a resolution of 400x400 pixels. The facilitators also limited facial disturbances from cosmetics, makeup, and headwear. This set of target photos and names included 50 local Israeli students. The photos were split between two groups of 25 each and distributed evenly among participants to keep set sizes manageable. Participants of study 1B included sixty-seven Israeli students (32 women, 35 men, all native speakers, M = 25.59 years [SD 3.36]). The procedure of study 1B age was similar to 1A, only instead of five name choices per picture, there were four. Eight of 1600 data points were discounted due to participant familiarity. The result of this study had an average success rate of 29.91%, with random chance at 25%. Study 1B replicated the face-naming effect while addressing the possible complication of external filler names in Study 1A. This replication was hypothesized to suggest that the face-name matching is true, and not solely a product of filler name features that cause them to stand out. (Ex. Name popularity or uniqueness.)
Study 2 replicated the face-naming effect in a different culture to determine if the effect
transcended cultures. It aimed to discount a theory that the effect appeared due to differences in target and filler names by employing a different method from study 1B. Following this, names were limited to French Caucasians to keep ethnicity constant. In this study, individuals emailed pictures owing to a lack of target faces. Study 2 included one hundred sixteen French participants (M = 23 years [SD 2.90]) The set of target names and photos consisted of French Caucasian age given names that were neither popular nor rare. The study was conducted similarly to the first two studies. The participants were shown a list of four names, with one being the true name. No data points were discounted due to zero participant familiarity with target faces. Participants matched names successfully at an average rate of 40.52%, with random chance at 25%. This success rate suggests that the face-naming effect is present in separate cultures.
Miller et al (2023) studied AI-Hyper-realism, specifically in AI-generated faces. In
Experiment 1, participants comprised 124 White US residents (62 women, neurotypical, M =
age 34.4 years, [SD = 8]). An assortment of one hundred AI-generated faces and one hundred human faces, all Caucasian (half male, half female), were used in the test. AI-generated faces were created using a StyleGAN2 model and matched with similar human faces. Participants were given approximately 100 assorted faces (half AI, half human), and asked to decide if a picture was human or AI-generated. Post-test, participants were asked to rate their confidence in their answer from 0-100, where 0 is not confident and 100 is completely confident. The study found that participants were more likely to select artificially generated faces as more human than human faces, and human faces were more likely to be judged as AI than AI faces. Participants most confident in their choice of ‘human face’ were more likely to choose an AI-generated face. In Experiment 2, there were 610 participants (312 women, M age = 35.3 years, [SD = 8.6 years]). Participants were asked to rate AI and human faces on one of 14 attributes. The study was blinded, and the participants were not informed that AI faces were present. Participant screening was otherwise identical to Experiment 1. Attributes tested included distinctiveness, attractiveness, familiarity, memorability, age, and nine other attributes commonly mentioned in reasoning during Experiment 1. Participants were found to describe AI faces as average, familiar,
attractive, and less memorable than actual human faces.
These two studies provide a groundwork for this study. Zwebner et al.'s (2017) study
analyzed the face-name matching effect, where participants had a significantly higher chance of guessing someone’s true name when provided with a photo of their face. This face-naming effect is instrumental in finding biases in participants and AI face generation. The face-naming effect was found to transcend culture, appearing in studies in both Israel and France. The following study aims to confirm the existence and accuracy of the face-naming effect via a group of participants comprising students and faculty from a Western Canadian High School. Miller et al.'s (2023) study on AI Hyper-realism provides evidence to support that AI-generated faces are nearly indistinguishable from human faces, especially those of Caucasian ancestry. This supports the assumption that participants generally cannot differentiate between AI and human faces.
Methods
Materials: filler names, AI-generated target faces, and target human faces and names.
Target names and matching human faces were collected. An assistant was directed to collect 20 faces and matching names from a popular social media platform. Since humans see AI-generated Caucasian faces as more human than other ethnicities (Miller et al, 2023), the assistant subjectively perceived the chosen faces as Caucasian or White. Since humans perceive Caucasian faces as more human, they should be more likely to have a matching human name. Although the pictures weren’t perfectly formatted with consistent lighting, the assistant collected photos where the target face takes up most of the image faces the camera and is generally well-lit. Subsequently, the assistant cropped these images to a square and assigned the target names to the corresponding faces. In six instances, the assistant removed the background due to the possibility of distraction. The filler names were then collected. Filler names are non-target names shown beside the target name and photo to provide a more diverse name selection. Each face was presented with three filler names along with the true name. The assistant gathered filler names from the closest three names on a name frequency chart (Statistics Canada Baby Name Observatory). The assistant was instructed to enter the target names in the field labelled ‘Select Name at Birth,’ keeping in mind the name’s frequency (based on an approximate age and binary
sex). A different name frequency chart was used for target participants born before 1993.
After finding the frequency of the name, the assistant selected three names with similar popularity. For example, if the target was a male born in 2000 named ‘Zachary,’ sample filler names would be ‘Tyler,’ ‘Ethan,’ and ‘Benjamin.’ The name was excluded if it was unusual or matched a separate target name. AI-generated faces were collected. A second study-blind assistant gathered these faces from a StyleGAN2 model and free-to-access website, named
“thispersondoesnotexist.com.” The assistant found Caucasian faces that matched the basic
categorization of the target human faces. The first assistant described the categorization of the human faces and gave these descriptions to the second assistant. Although there was some deviation in collecting the faces, the photos collected all faced the ‘camera’ and were well-lit. The selection guidelines were based on age, gender, and hair. The age categories were: young adult (~18-30), adult (~30-45), middle age (45-60), and senior (~60+). The gender categories were basic, only generalized and subjectively judged as ‘male’ and ‘female.’ Hair categories consisted of ‘head-hair’ (fully bald, half-bald, short, medium, long), and facial hair (clean-shaven, mustache, beard). The assistant assigned the StyleGAN2 photos to the same true and filler names as the human face.
Procedure
This study had two testing sections. The first section was a control group, to establish the
face-naming effect in a Canadian context. This section was a close recreation of Zwebner et al.'s (2017) 1A study. The control group used human faces, target names, and filler names. Each photo was printed onto a piece of paper, with the target and filler names ordered randomly beneath it, and labelled a, b, c, d. Each participant was given a stapled booklet containing the pictures and corresponding names (Example to the Left) and a
separate paper to input their name selection. The participants
were instructed to look at the photo on the paper and select any of
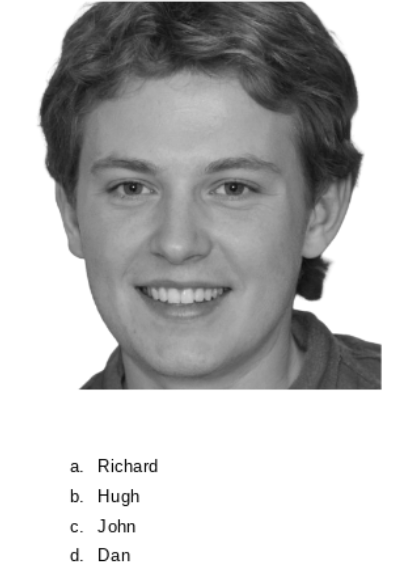
the four names that they thought were the true names of that
person. They were instructed to tell the facilitator if they were
familiar with or recognized any of the faces. In the second
section, StyleGAN2 faces became a factor. This section aimed at
testing if AI-generated headshots had the same name as their
counterpart and if the face-naming effect was due to basic
features or other factors. The main procedure was the same as the first section, however, minor changes were made. The AI-generated faces were randomly ordered in the booklet given to participants. The same instructions as section two were given to participants.
Participants
Before the experiment started, participants completed a demographic survey. The survey
was designed to find basic information, such as culture, ethnicity, and identity. The survey is below.
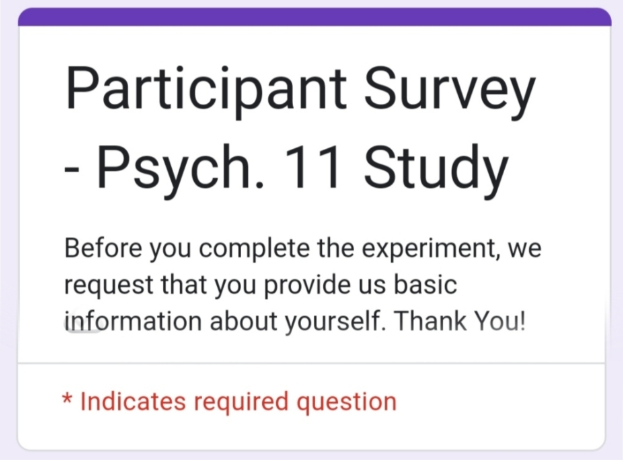
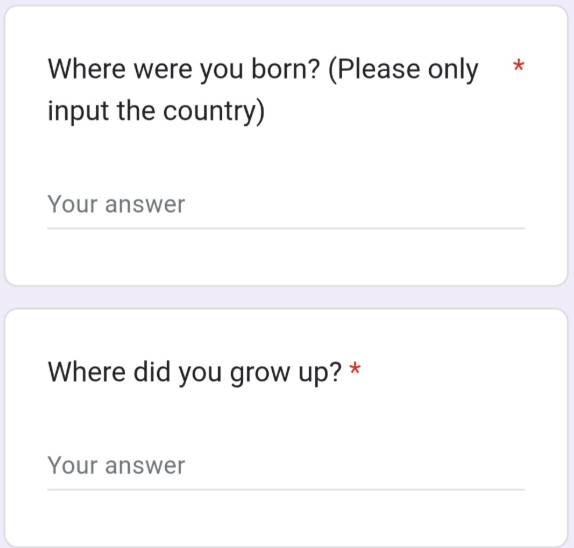


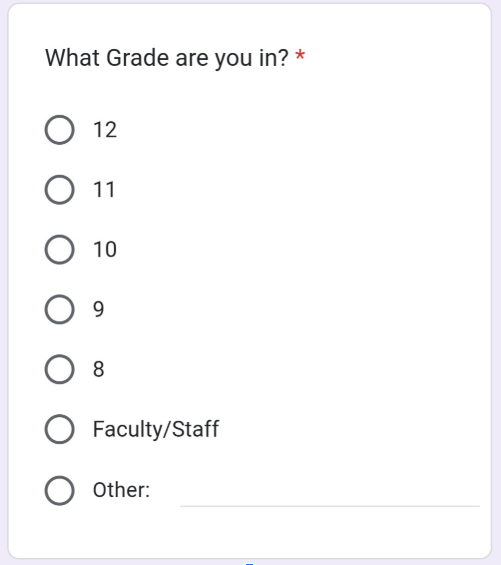
After this survey, participants were asked to
complete the booklet (as described above in
“Procedures.”). Post-completion of the
experiment, participants were compensated with
assorted candy and chocolate.
Data
Age
Grade | 8 | 9 | 10 | 11 | 12 | Staff |
Participants | 1 | 4 | 1 | 5 | 11 | 1 |
Nationality
Country | Canada | USA | Germany | Thailand | Philippines |
Participants | 18 | 2 | 1 | 1 | 1 |
Gender
Gender | Male | Female |
Participants | 15 | 7 |
Religion
Religion | None | Christian | Agnostic | Buddhist | Unique |
Participants | 10 | 7 | 4 | 1 | 1 |
Results
Each percentage/score is based on the participant's score in the experiment, out of 20. For
example, if a participant scored 4/20, their accuracy would be 20%.
Varied (AI) Experiment (%) | Control (Human) Experiment (%) |
Mean = 17.81%, Median = 20% | Mean = 19.48%, Median = 17.5% |
Trial 1 Results
Varied (AI) Experiment (/20) | Control (Human) Experiment (/20) |
2 | 1 |
3 | 3 |
3 | 4 |
4 | 4 |
4 | 4 |
5 | 5 |
6 | 6 |
6 | 6 |
Mean = 4.125 (20.625%), Median = 4 (20%) | Mean = 4.125 (20.625%), Median = 4 (20%) |
Trial 2 Results
Varied (AI) Experiment (/20) | Control (Human) Experiment (/20) |
1 | 4 |
3 | 4 |
3 | 3 |
5 | |
Mean = 3 (15%), Median = 3 (15%) | Mean = 3.67 (18.33%), Median = 4 (20%) |
Limitations
This study contained twenty-three participants. In Zwebner et al.’s (2017) studies, their
participant yield was significantly greater, all involving more than one hundred participants.
Therefore, this study was not replicated. In this study, participants had a high demographic
diversity, whereas, in Zwebner et al.'s (2017) study, participants were generally of a single
cultural demographic.
Discussion
Participants comprised a sample of students from Grades 8 to 12, as well as a single data
point from a school faculty member. Overall, the study had 23 participants (1 faculty member, 22 students). Of these students, the mean score was 10.96 and the median grade was 12 (11.5 rounded), with half the student data points coming from grade 12. The distribution of student participants' ages is presented in the table (Right).
Within these grades, there was some variance in Nationality, Religion, and Gender. The majority of participants were Canadian, with 78.3% self-reporting Canadians. 95.6% of participants reported that they grew up in or in combination with Canada. For Religion, 43% stated they have no religion, 30.4% were Christian, 17.4% were Agnostic, and 8.6% reported another religion/belief. Among the participants who reported a Gender, 31.81% were Female, and 68.18% were Male. In the first trial, there were 16 participants (Below). Since both the control and varied experiments were conducted at the same time, half of the
participants completed the control (human-face experiment),
and a half completed the varied (AI-faces experiment). In this Trial, Participants were instructed to complete the demographic survey first, and then complete the experiment.
Grade | 8 | 9 | 10 | 11 | 12 |
Participants | 1 | 4 | 1 | 5 | 11 |
Grade | 8 | 9 | 10 | 11 | 12 | Staff |
Participants | 0 | 4 | 0 | 2 | 9 | 1 |
In the second trial, there were seven participants (Below, Left).
Grade | 8 | 9 | 10 | 11 | 12 |
Participants | 1 | 0 | 1 | 3 | 2 |
Nearly half the participants completed the human-face experiment, and over half completed
the AI-face experiment (3:4, respectively). In this trial, participants were instructed to complete the demographic survey first and then complete the experiment. Overall, participants in the human-face experiment scored an average of 19.48%, and participants in the AI-face experiment participants scored an average of 17.81%. In both experiments, the chance of naming a face correctly was 25%. In both the human-face experiment and the AI-face experiment, participants on average scored lower than random chance.
In the human-face experiment, participants scored a median of 20%, and in the AI-face experiment, participants scored a median of 17.5%. In the human-face experiments, the most common accuracy was 20%, and in the AI-face experiments, it was 15%. In each experiment, two participants scored at an accuracy above random chance, all with an accuracy of 30%.
For eighth grade, the result was 15%, having only one data point. Ninth grade had 4 data
points, which were equally distributed between human and AI-face experiments. The accuracy in the human-face and AI-face experiments was 20% and 22.5%, respectively. The grade 10 results had only one data point at 15%. In the Grade 11 results, the accuracy was 17.5% for the human-face experiment, and 13.34% for the AI-face experiment (Median = 15%).
The Grade 12 group achieved an accuracy of 20% in the human-face experiment, and 20.83% in the AI-face experiment. The median accuracy is 20% and 22.5%, respectively. The Staff group had only one data point at 30% accuracy in the human-face experiment.
Of the five religion-based groups considered in this experiment, the maximum belonged
to the ‘No religion’ group. This group had an accuracy of 23% in the human-face experiment,
and 21% in the AI-face experiment. The median accuracy was 20% and 25%, respectively. In the Christian group, there was an accuracy of 20% in the human-face experiment, and 17% in the AI-face experiment. The median accuracy is 20% and 15%, respectively. Within the Agnostic group, the accuracy was the same for both experiments, at 17.5%. The Buddhist group had only one data point, which was from the human-face experiment at 15%. Similarly, the unique beliefs group had only one date point at 15% from the human-face experiment.
Only male and female genders were discussed in this study. The Male group had
an accuracy of 18.75% in the human-face experiment, and 15.71% in the AI-face experiment.
The median accuracy for Male participants was 20% and 15%, respectively. The Female group achieved an accuracy of 23.33% in the human-face experiment and 22.5% in the AI-face experiment. The Median accuracy for Female participants was 20% and 22.5%, respectively.
Conclusion
The joint aims of this study were to test the ‘face-naming’ effect and the interactions of
facial features and characteristics within a mid-sized high school in a small town near Vancouver, BC (largely comprising people of European descent). The results deviated from Zwebner et al.’s (2017) study, possibly due to different naming conventions and local culture compared to the French and Israeli participants in said study. The second hypothesis was that facial characteristics affect the face-naming effect. From the data, this is weakly supported. The mean accuracy of participants in the AI-faces experiment is approximately 17.8%, which is ~1.7% lower than the accuracy of participants in the human-faces experiment. This could show a disconnect between exact facial characteristics and the face naming effect. The greatest factors that influence the accuracy of the face-naming effect are the culture and gender of the participant and the culture of the person whose name is guessed.
Suggestions for Future Research
Future must validate the results obtained using large random samples. Studies should be
conducted in environments with different specific population demographics, such as a
comparative analysis of conservative and liberal populations. This would increase the reliability of the results and show the impacts of political and social impacts through the differences in the usage of family names v. names new to a lineage. The study should include more female participants. The data obtained through this research may suggest that females have a much higher accuracy than that of male participants. The methods employed for the collection of human faces must be improved with more consistency in lighting, positioning, and emotion. The quality of AI-generated faces must be streamlined and improved with strict descriptions and more similarities in facial structure, body typing, or secondary ethnicity.
Written By: Levi Cottrell
Works Cited
(1) Miller, E. J., Steward, B. A., Witkower, Z., Sutherland, C. A. M., Krumhuber, E.G., &
Dawel, A. (2023, November 13). AI Hyperrealism: Why AI Faces Are Perceived as More
Real Than Human Ones. journals.sagepub.com. Retrieved October 7, 2024, from
(2) Zwebner, Y., Sellier, A.-L., Rosenfeld, N., Goldenberg, J., & Mayo, R. (n.d.). We Look
Like Our Names: The Manifestation of Name Stereotypes in Facial Appearance.
APA.org. Retrieved Sept. 26, 2024,
Comments